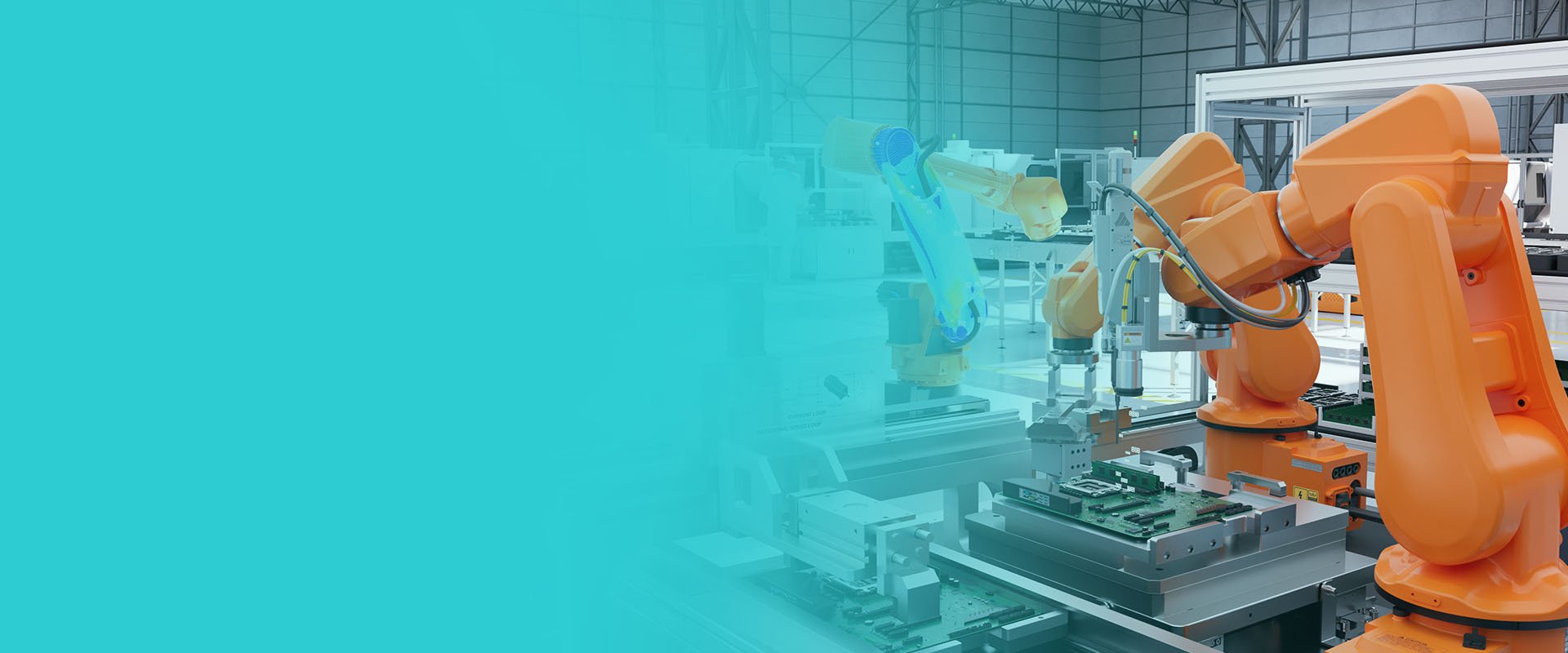
製造業界のためのデジタルツインテクノロジー
あらゆる製造プロセスのゴールは、高品質な製品の設計と製造です。業界最高水準の シミュレーション、人工知能(AI)、データ分析、ハイパフォーマンスコンピューティング(HPC)ソリューションを備えたアルテアの包括的なデジタルツインソリューションにより、企業やチームは、イノベーションを引き出し、時間を節約し、無駄を最小限に抑える比類なきエンドツーエンドの力を得ることができます。
アルテアのデジタルツインソリューションは、バーチャル試運転、シミュレーション技術、デジタルスレッドの統合、リアルタイムの資産管理により、製造分野を支援します。バーチャル試運転とシミュレーション機能を活用することで、製造システムを構築する前に最適化し、コストの削減とリスクの最小化を図ることができます。 さらに、製品ライフサイクルを通じてデジタルスレッドをシームレスに統合することで、リアルタイムの資産監視と管理が可能になり、メンテナンススケジュールの最適化と設備の改善が実現します。
製造分野におけるアルテアのデジタルツイン機能は、軽量化、トポロジー最適化、マルチフィジックスシミュレーション、モデルベース開発なども包含しています。世界のデジタルツインに関する動向は、2022年Altairデジタルツイン世界調査報告書でご覧いただけます。
製造業デジタルツインインサイト
Altairのテクノロジーと専門スタッフが、みなさまのデジタルツインのスタートをお手伝いします。
お問い合わせ製造業向けデジタルツインセントラル
デジタルツインの活用

Digital Twin for Sustainable Energy
Improving lifetime value of a fusion powerplant using a physics-based digital twin. Assystem is an international engineering and digital services group focused on low-carbon projects that accelerate the transition to clean energy. Assystem is committed to the development of decarbonized electricity (fusion energy, renewables and electricity grids) and clean hydrogen. The Assystem team wanted to leverage the expensive design models to create a digital twin by inputting the sensor data that was livestreamed from the plant, which helps engineers understand the plant's structural integrity and further optimize inspection and maintenance schedules.
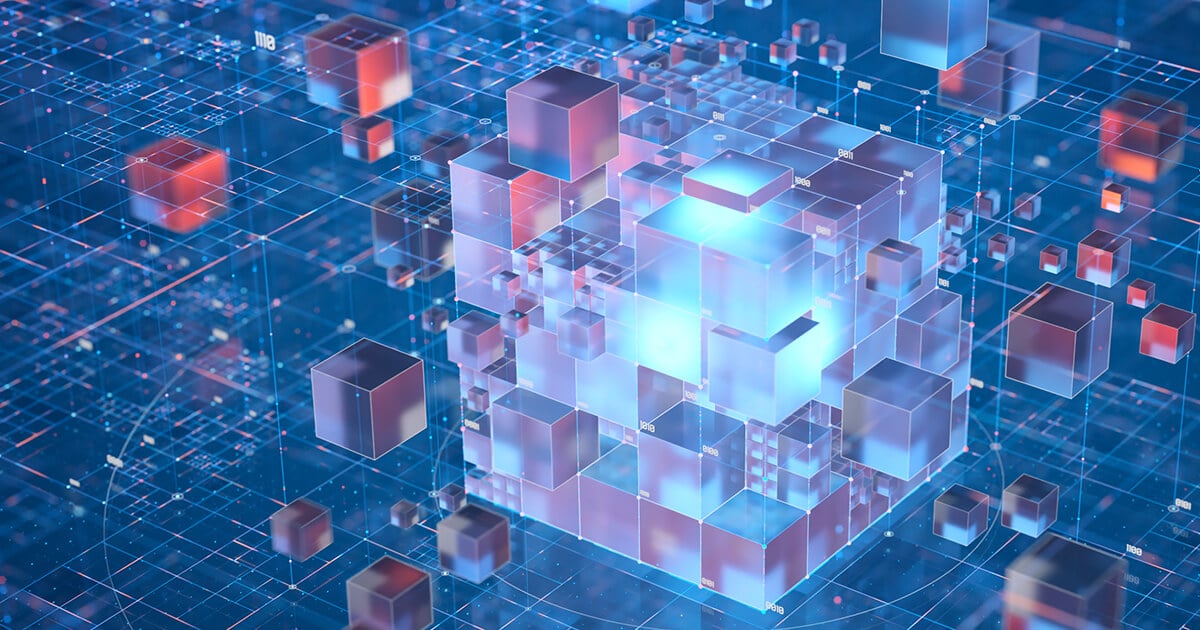
Digital Twin Implementation: A Strategic Tool to Improve Process/Product Capabilities in Sheet Metal Forming
Sheet Metal Forming has different and wide industrial applications (Automotive, White Goods, Aeronautic, etc....). In the metal forming industry, the simulation of processes and the resulting material behavior is of high importance. Important process parameters (e.g., material flow, temperature range, force required), as well as the resulting material characteristics (e.g., strength, residual stress, temperature resistance), can be supported using FEA to replace costly and uneconomical practical tests.
The re-use of knowledge gained from these FEA simulations in combination with data provided by different sensors is the next step towards the implementation of a Digital Twin. Its integration into the IT architecture of a digital factory is inevitable to increase the efficiency and environmental sustainability of processes and products in manufacturing. Therefore the presented project relies on Reduced Order Models in use of Machine Learning approaches as well as an IoT-based dashboard for the combined visualization of actual data and derived KPIs. As a result, the implemented solution enables significant improvement of capabilities in the considered context.
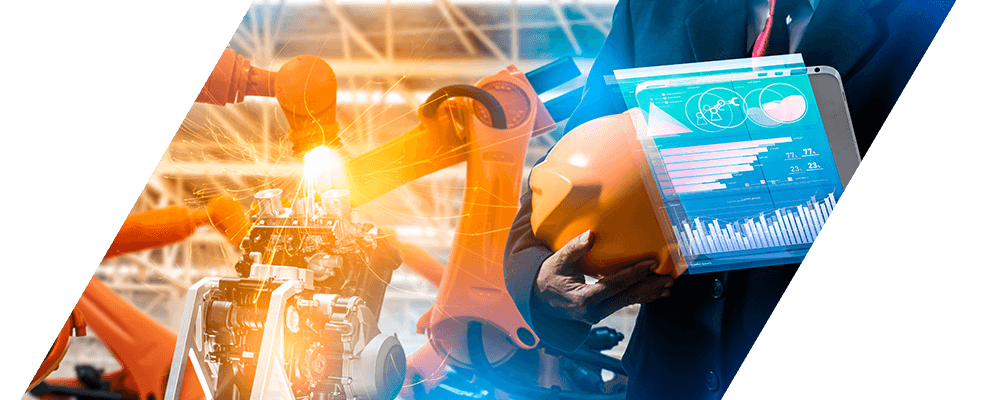